Feasibility study explored the potential of using Artificial Intelligence to identify possible concerns
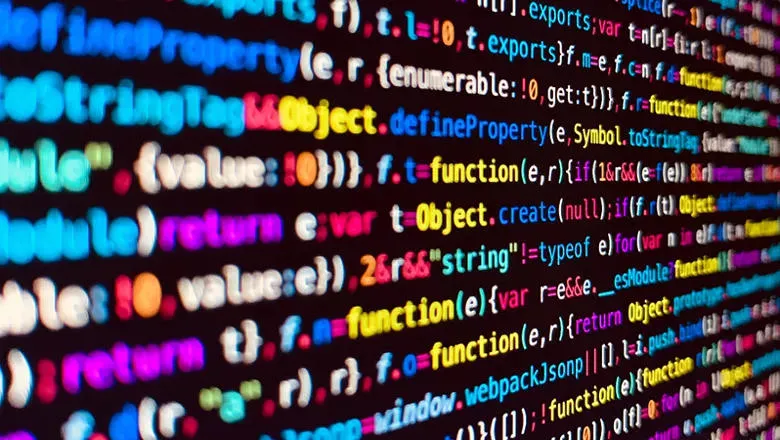
This research, working closely with people with lived experience and other stakeholders, demonstrated that there are opportunities to better use data and explored the potential of using Artificial Intelligence to identify possible concerns.
The abuse of people with a learning disability and autistic people sadly features in several health and care services across the years. Researchers have consistently reported that people with a learning disability and autistic people are more at risk of abuse than other groups, especially when living in residential services (as compared to older people more generally, or those people with mental health needs).
In England care homes and supported living providers who provide personal care must be registered, meet set standards, and are inspected by the regulator, the Care Quality Commission (CQC). Its role is to register health and care providers as well as monitor, inspect and regulate health and adult social care services. The CQC collects information from and about these services and visits them to see evidence and to talk to residents, their families and staff through its regulatory role and inspection process.
Today's report presents findings of an independent study exploring the potential for using data to predict and detect abuse in care homes and supported living services for people with a learning disability and autistic people. It builds on suggestions that there may be scope to use Artificial Intelligence (AI) to interrogate the CQC's data efficiently and effectively and reflects wider debates about the potential for using AI to serve the needs of society.
This research, funded by the National Institute for Health and Care Research, demonstrated the feasibility of researchers accessing CQC data for analysis and the considerable amount of information potentially useful in the prediction and detection of abuse in care homes and some supported living settings. It also revealed that the analytic potential of these data would be enhanced through its greater digitisation and that having this information in analysable form at service level could be helpful in developing risk indicators and identifying trends in abuse across services.
The study also highlighted known gaps in information relating to supported living services.
Natural Language Processing (NLP) gives the opportunity to harness the potential of some of CQC's data. However, when using such methods, this research identified that it is important not to lose the 'human perspective' and that the contribution of public contributors and other stakeholders in research using NLP and in the CQC's work more widely is essential.
The report identifies six recommendations for future research and concludes that:
"there is appetite for enabling regulatory and inspection systems and processes to benefit from advances in information processing, such as AI, and, thereby, potentially to prevent or respond to the abuse and neglect of people living in care homes and supported living services. Our focus is on services in England for adults with a learning disability and autistic adults within the context of the regulatory regime of the Care Quality Commission (CQC), but our observations may be more widely applicable. Our study took place at a time of change of activities within the CQC and the passing of a new law related to supported living services; such times can provide opportunities to do things differently." (p.8)
The research revealed the complexities of using data to help predict and detect abuse in care homes and supported living services. It argues "whilst some of these complexities could be addressed within the existing regulatory and legislative context, many of the issues underlying this require an urgent wider multi-departmental central government policy response" (p.58). This includes the oversight of supported living arrangements.
Acknowledgements and disclaimer
This report presents independent research funded by the National Institute for Health and Care Research (NIHR) Policy Research Programme (Policy Research Unit in Health and Social Care Workforce: Ref. PR-PRU-1217-21002). The views expressed here are those of the authors and not necessarily those of the NIHR or the Department of Health and Social Care.
We are extremely grateful to the public contributors and stakeholders who shared their views and experiences with us; their input and support were invaluable. Also, for the assistance and support of colleagues within the Care Quality Commission who facilitated access to the data and shared their extensive knowledge of data collections with us. The study was also discussed with the Unit's Patient and Public Involvement and Engagement Advisory Group who provided valuable comments, especially Andrew Carpenter, who also reviewed and commented on the summary of this report. Thanks are also due to Sam Rickman, for sharing with us his helpful insights into the application of machine learning and natural language processing to adult social care data in the early stages of the project.
This publication
Chester, H., Martineau, S., Manthorpe, J., Hickman, B., Cooper, V. and Yuan, Z. (2024) Predicting and detecting abuse in registered care homes and supported living for people with a learning disability and autistic people, London, NIHR Policy Research Unit in Health and Social Care Workforce, The Policy Institute, King's College London. https://doi.org/10.18742/pub01-166
See also the accompanying Easy Read Summary Report.