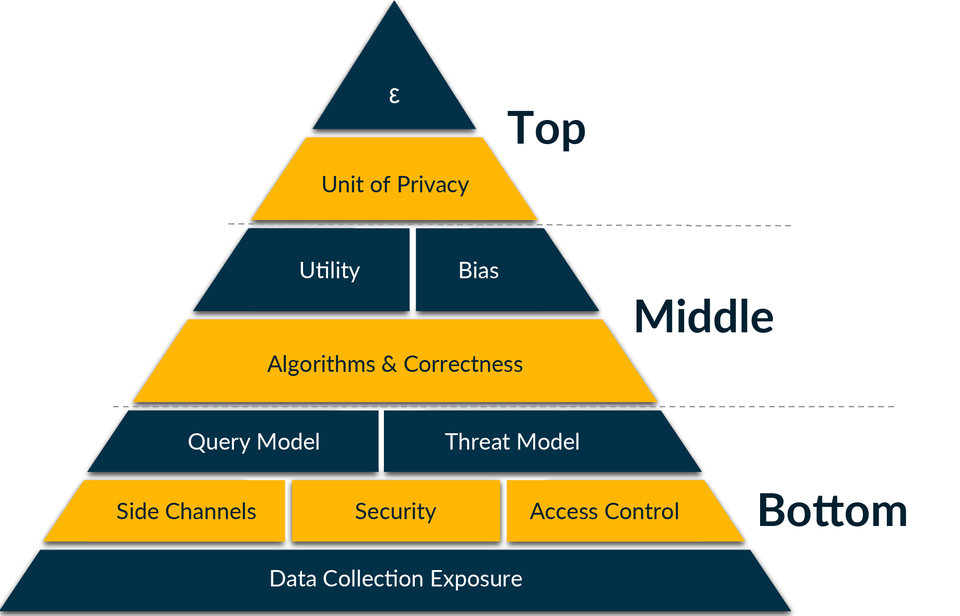
Evaluating any claim to differential protection requires examining every component of the differential privacy pyramid. Its top level contains the most direct measures of privacy guarantees, including epsilon, which is a numerical value of how strong the privacy guarantee is. The middle level includes factors that can undermine a differential privacy guarantee, such as lack of sufficient security, and the bottom level includes underlying factors, such as the data collection process. The ability for each component of the pyramid to protect privacy depends on the components below it.
NIST
Here's a tricky situation: A business that sells fitness trackers to consumers has amassed a large database of health data about its customers. Researchers would like access to this information to improve medical diagnostics. While the business is concerned about sharing such sensitive, private information, it also would like to support this important research. So how do the researchers obtain useful and accurate information that could benefit society while also keeping individual privacy intact?
Helping data-centric organizations to strike this balance between privacy and accuracy is the goal of a new publication from the National Institute of Standards and Technology (NIST) that offers guidance on using a type of mathematical algorithm called differential privacy. Applying differential privacy allows the data to be publicly released without revealing the individuals within the dataset.
Differential privacy is one of the more mature privacy-enhancing technologies (PETs) used in data analytics, but a lack of standards can make it difficult to employ effectively - potentially creating a barrier for users. This work moves NIST toward fulfilling one of its tasks under the recent Executive Order on AI: to advance research into PETs such as differential privacy. The order mandates the creation of guidelines, within 365 days, to evaluate the efficacy of differential-privacy-guarantee protections, including for AI.
While NIST's new guidance, formally titled Draft NIST Special Publication (SP) 800-226, Guidelines for Evaluating Differential Privacy Guarantees, is designed primarily for other federal agencies, it can be used by anyone. It aims to help everyone from software developers to business owners to policy makers understand and think more consistently about claims made about differential privacy.
"You can use differential privacy to publish analyses of data and trends without being able to identify any individuals within the dataset," said Naomi Lefkovitz, manager of NIST's Privacy Engineering Program and one of the publication's editors. "But differential privacy technology is still maturing, and there are risks you should be aware of. We want this publication to help organizations evaluate differential privacy products and get a better sense of whether their creators' claims are accurate."
The need for understanding of differential privacy and other PETs is pressing, in part because of the rapid growth of artificial intelligence, which relies on large datasets to train its machine learning models. Over the past decade, researchers have demonstrated that it is possible to attack these models and reconstruct the data they were trained on.
"If it's sensitive data, you don't want it revealed," Lefkovitz said. "We learned in our recent U.S.-U.K. PETs Prize Challenges that differential privacy is the best method we know of for providing robust privacy protection against attacks after the model is trained. It won't prevent all types of attacks, but it can add a layer of defense."
As an idea, differential privacy has been around since 2006, but commercial differential privacy software remains in its infancy. Prior to this publication, NIST created an introductory blog series designed to help business process owners and privacy program personnel understand and implement differential privacy tools available in NIST's Privacy Engineering Collaboration Space.
This new publication is an initial draft, and NIST is requesting public comments on it during a 45-day period ending on Jan. 25, 2024. The comments will inform a final version to be published later in 2024.
As the publication's title implies, it has been challenging to evaluate the claims of differential privacy software makers. A typical promise, or guarantee, that a manufacturer might make is that if its software is used, an attempt to re-identify an individual whose data appears in the database will be unsuccessful.
Evaluating a real-world guarantee of privacy requires an understanding of multiple factors, which the authors identify and organize graphically in a "differential privacy pyramid." The ability for each component of the pyramid to protect privacy depends on the components below it, and evaluating any claim to differential privacy protection requires examining every component of the pyramid. Its top level contains the most direct measures of privacy guarantees; the middle level includes factors that can undermine a differential privacy guarantee, such as lack of sufficient security; and the bottom level includes underlying factors, such as the data collection process.
One of the main points of the publication, Lefkovitz said, is to make this technical topic comprehensible to users who may not have technical expertise.
"We show the math that's involved, but we are trying to focus on making the document accessible," she said. "We don't want you to have to be a math expert to use differential privacy effectively."
Comments on the draft guidelines are due by Jan. 25, 2024. To submit comments, download the template from the NIST website