SpatialData is a freely accessible tool to unify and integrate data from different omics technologies accounting for spatial information, which can provide holistic insights into health and disease
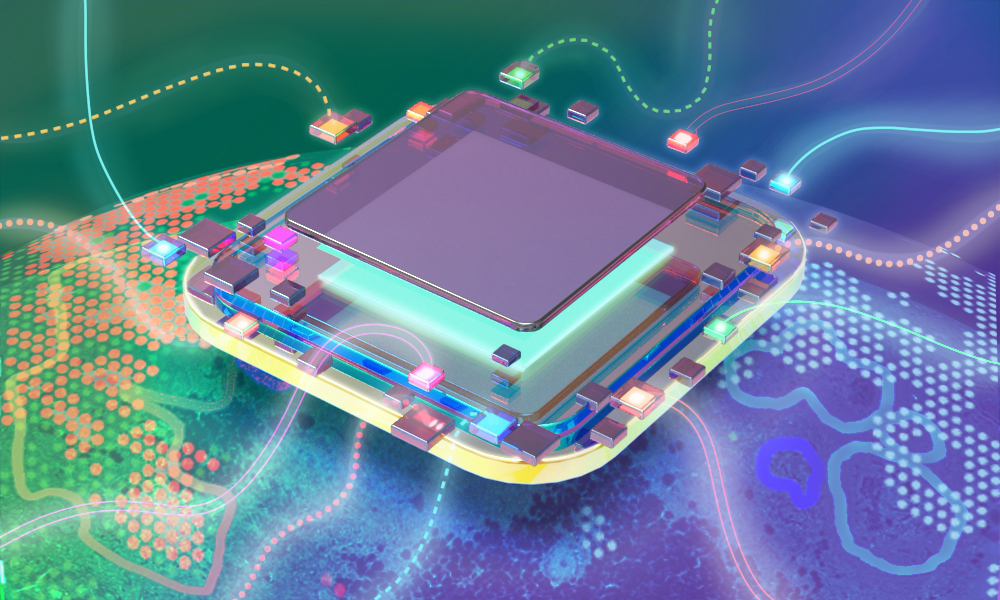
Written by Luca Marconato, Kevin Domanegg and Lisa Vollmar
Biological processes are framed by the context they take place in. A new tool developed by the Stegle Group from EMBL Heidelberg and the German Cancer Research Centre (DKFZ) helps put molecular biology research findings in a better context of cellular surroundings, by integrating different forms of spatial data.
In a tissue, every individual cell is surrounded by other cells, and they all constantly interact with each other to give rise to biological function. To understand how tissues work or malfunction in diseases such as cancer, it is crucial to not only learn the characteristics of every cell, but also account for their spatial context. Quantitative characterisation of cells in the context of the physical space they inhabit is key to understanding complex systems.
The technologies enabling these types of exploration are called spatial omics technologies, and their progressing development is contributing to the rise in popularity of spatial biology. Such technologies can give detailed information about the molecular makeup of individual cells and their spatial arrangement. However, these technologies focus on different characteristics of a cell - such as RNA or protein levels, and the resulting datasets are managed and stored in diverse ways. To solve this challenge, a collaborative project led by the Stegle Group developed SpatialData, a data standard and software framework which allows scientists to represent data from a wide range of spatial omics technologies in a unified manner.
Technology development for spatial biology
Over the last decade, numerous technologies have been developed by both academia and industry for spatially visualising tissues, cells, and subcellular compartments. However, each technique focuses on a small number of desirable characteristics and presents related trade-offs. For instance, Visium from 10x Genomics captures information about the expression of all genes in a tissue, but does not provide single-cell resolution. In contrast, the 10x Genomics Xenium assay, MERFISH, or the MERSCOPE platform from Vizgen yield fine-grained maps of gene expression with subcellular resolution. However, these assays are currently limited to a few hundred preselected genes. And the list of such technologies, each providing a small slice of the full picture, keeps growing.
Challenges of spatial omics technologies
This heterogeneity of technologies is reflected on the computational side by an even greater heterogeneity of file formats: each technology comes with its own storage format, and often data generated by the same technology can be stored in multiple formats.
Practically, this brings several challenges to the analysis of spatial omics data. Visualisation and analysis methods are usually tailored to a specific technology, which limits data compatibility and makes it hard to integrate different methods into a single analysis pipeline. However, for a holistic understanding of a biological system, it's important to simultaneously look at different cell characteristics or samples from different locations. Omics technologies generate enormous amounts of data (terabytes of images, millions of cells, billions of single molecules), demanding optimised engineering solutions. Hence, spatial biology urgently needs a universal framework that can integrate data across experiments and technologies, and provide holistic insights into health and disease. This is where SpatialData steps in.
SpatialData - a framework to unite them all
"There is a strong need to establish community solutions for the management and storage of spatial omics data. In particular, there is a need to develop new data standards and computational foundations that allow for unifying analysis approaches across the full spectrum of different spatial omics technologies that are emerging," said Oliver Stegle, Group Leader at EMBL in the Genome Biology Unit, and head of the Computational Genomics and Systems Genetics division at the German Cancer Research Center (DKFZ). "A first major step in this direction is SpatialData, a data standard and software framework that bridges and adapts previous data management concepts from single-cell multi-omics to the spatial domain."
SpatialData unifies and integrates data from different omics technologies, bridging state-of the-art-technologies with a framework that allows for computationally performant access and manipulation of the data. This tool was introduced in a recent Nature Methods publication, authored by Luca Marconato during his PhD at EMBL in the Stegle Group, a joint degree with the Faculty of Bioscience of the University of Heidelberg. "We developed the SpatialData framework to alleviate the data representation challenges when studying spatial biology, so that the researcher can focus on the biological analysis, rather than being slowed down by tedious data manipulations, otherwise required to even just visualise the data. The framework provides a unified representation and implements ergonomic operations for convenient processing of spatial omics data." said Marconato.
The tool enables any researcher to import their data and perform tasks like data representation, processing, and visualisation. Additionally, it gives the option to interactively annotate the data, and save it in a language-agnostic format, facilitating the emergence of analysis strategies that combine methods from different programming languages or analysis communities.
The framework has been developed as a collaborative project between multiple institutions such as the DKFZ, the Technical University of Munich, the Helmholtz Centre Munich, German BioImaging, the ETH Zürich, VIB Center for Inflammation Research in Belgium, as well as the Huber and Saka groups at EMBL.
"We have conducted our research and technological development keeping the benefit for the bigger science community in mind", said Giovanni Palla, co-first author and PhD student at the Helmholtz Centre Munich. "We not only established an interdisciplinary collaboration project between research institutes but also worked closely with developers working with different spatial technologies and in different programming languages to address the problem of interoperability. As a result, our framework is compatible with the vast majority of spatial omics assays from academia and industry. Being published openly, other researchers can now freely use SpatialData to manage their own data and have the opportunity to collaborate across various technologies and research topics."
"In our paper, we illustrate three important features of SpatialData," explained Kevin Yamauchi, co-first author and a postdoctoral researcher at ETH Zürich. "First, we present a standardised interface and unified storage format (based on the OME-NGFF) for all spatial omics technologies. Second, using the unified representation, we integrate signals from multiple modalities. Here, we transfer annotations across modalities and quantify signals using these transferred annotations. Finally, we present a way to interactively annotate (pathology) images and use the annotations to analyse the associated molecular profiles."
SpatialData provides an interactive representation of data, both on your hard drive and your computer's RAM, which enables the analysis of large imaging data or multiple geometries or cells. Other prominent key features are the framework's ability to align and annotate omics data in a common coordinate system. Thus, SpatialData enables the efficient management and manipulation of spatial datasets, including the definition of a common coordinate system across sequencing- and imaging-based technologies.
Application in breast cancer
The interdisciplinary team used the SpatialData framework to reanalyse a multimodal breast cancer dataset from 10X Genomics as a proof of concept. This dataset comprises consecutive sections of the same breast cancer block, where each section is analysed using different technology, like Visium, Xenium, and a separate scRNA-seq dataset. The study demonstrates the complementary nature of these technologies. "By integrating 10X Xenium and scRNAseq, we mapped the cell types into the space," said Elyas Heidari, a PhD candidate at DKFZ and one of the authors of the study. "Next, we used 10X Visium to identify cancer clones in space. This can be done because we have transcriptome-wide readouts. Finally, we used the H&E stained microscopy images to identify regions of interest for histopathology annotations. This analysis successfully showcased a unique application of SpatialData in unlocking multi-modal analyses of spatially-resolved datasets."
In the future, a patient's tumour might be analysed with different technologies commonly used in the clinic, with the data then unified bySpatialData to gain a holistic understanding of the tumour. Furthermore, the interactive interface would allow the doctor to annotate the data, thus enabling detailed analysis of specific tumour regions and characteristics, potentially leading to personalised treatment approaches.