Researchers in the UC Davis HealthDepartment of Radiology have developed a new way to use EXPLORER, the total-body positron emission tomography (PET) scanner, to speed PET parametric imaging that can detect cancer.
The new approach, called relative Patlak plot, shortens the overall scan time, making it easier for patients to tolerate the scan. The study was published in the Journal of Nuclear Medicine.
"Parametric PET imaging for cancer often provides better lesion contrast and quantification than conventional static PET imaging, but it typically requires a time of up to one hour, which poses challenges for widespread use," said Guobao Wang, professor of radiology and senior author of the paper. "However, with the method detailed in the paper, we can now make parametric imaging feasible for routine clinical PET scans in 20 minutes or less."
To detect and determine the stage of a cancer, EXPLORER reads how radioactive glucose tracers are distributed in a patient's body. Glucose tracers are special sugar molecules with a tiny "tag" on them that lets doctors or scientists see where the sugar goes in the body. Because cancers have higher metabolic needs, the radioactive glucose tends to concentrate in tumors, lighting them up for the scanner.
Parametric PET imaging is better at tracking tracers in the body and highlighting the metabolic processes guiding their movement. Patlak plots are a commonly used model but require a lot more scanner time than static PET imaging.
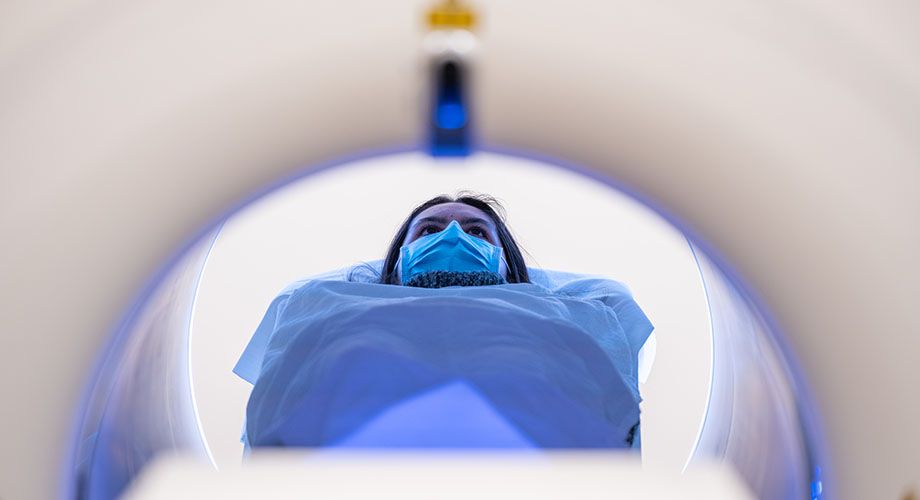
EXPLORER was invented at UC Davis and was the first clinically approved total-body PET scanner in the world.
In the study, the research team tested relative Patlak plots, a new variation on the older model, in 22 human participants: 12 healthy and 10 with cancer using EXPLORER, invented by UC Davis and manufactured by United Imaging Healthcare. They found the new method produces excellent results, similar to the original, but takes a lot less time to gather data.
The most significant problem the team had to address with relative Patlak plots was images that are grainy and harder to read. To overcome this issue, Siqi Li, assistant project scientist in radiology and first author on the paper, used an artificial intelligence technique called "deep kernel noise reduction." It combines deep learning and kernel methods (which help algorithms manage non-linear data). This significantly reduced the data noise, providing excellent images.
"We found the relative Patlak method is both feasible and accurate," said Li. "Our images showed excellent lesion contrast and allowed us to better visualize the heart. These improvements open up opportunities to further explore the benefits of parametric PET imaging."
Li has recently received a grant to further develop this method. He is also collaborating with Lorenzo Nardo, director of Nuclear Medicine, and Marcio Malogolowkin, chief of Pediatric Hematology-Oncology, to expand these advances to pediatric cancer patients. Other co-authors included Yasser Abdelhafez, Simon Cherry and Ramsey Badawi, all from UC Davis.