
Same density, different patterns and cancer risk. As seen with mammography, breasts with the same density can have different texture patterns (seen above). In a new study, patterns of breast tissue apparent on mammograms and quantified by computer analysis were a better predicter of breast cancer risk than breast density. Images provided by Despina Kontos.
A mammogram can reveal whether a woman has dense breasts, a feature linked to a higher risk of breast cancer, and needs extra screening.
A new study now suggests that six different breast texture patterns, also seen in mammograms, can do a better job at predicting which women will develop breast cancer in the future.
A computer algorithm, developed by Columbia University and Mayo Clinic researchers, that detects and quantifies the patterns could soon be added to digital mammography systems to provide clinicians with better risk estimates.
"These tools will pave the way for personalized screening recommendations and better management of women's health care, reducing the frequency of screening for low-risk women and increasing the frequency for women who need it," says study co-leader Despina Kontos, the Herbert and Florence Irving Professor of Radiological Sciences at Columbia University Vagelos College of Physicians and Surgeons.
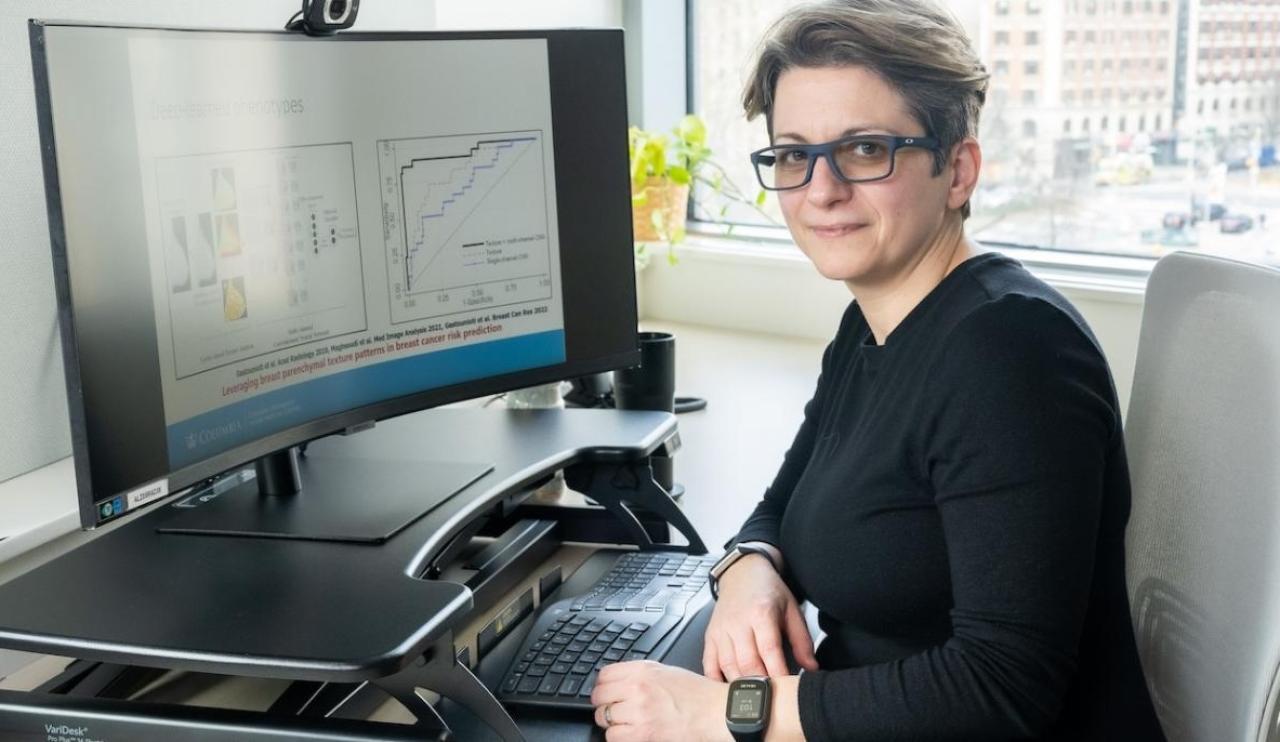
Despina Kontos
"They may also help us do a better job of identifying women who would benefit from interventions to lower their risk of breast cancer."
Since the adoption of digital mammography about two decades ago, computer scientists like Kontos have been looking to capture details from mammograms that go beyond breast density and can better predict individual risk.
Smaller, earlier studies suggested that differences in texture-patterns in the way fat and glandular tissue is distributed in the breast-may also be related to breast cancer risk.
"Different patterns are visible to radiologists who examine mammography images-some look clumpy, some look smooth, some look more spotty," Kontos says. "But beyond making a determination of overall density, radiologists had no way to quantify these patterns and turn them into data needed to determine their link to cancer risk."
The new study, one of the largest of its kind, used an automated computerized approach to identify and quantify different breast texture patterns in mammography images from over 30,000 women. The researchers then examined the mammograms of 3,500 women, some of whom were later diagnosed with breast cancer, to see if the patterns were associated with higher rates of breast cancer.
Six breast texture patterns were found to be associated with greater breast cancer risk in both white and Black women.
"Given that women with the same breast density can have substantially different texture patterns, our analysis provides important additional information for estimating breast cancer risk," Kontos says."Given that women with the same breast density can have substantially different texture patterns, our analysis provides important additional information for estimating breast cancer risk."
Additional data, including genetics and lifestyle information, are being included in the model to see if its predictive power can be increased further. Kontos and her collaborators are also experimenting with AI approaches that may reveal predictors currently hidden in the mammography data and extending their analysis to 3D mammography images.
"Screening is cornerstone of early detection," Kontos says. "Improving its ability to make predictions will increase its role in breast cancer prevention even further."
References
More information
The study, "Radiomic Parenchymal Phenotypes of Breast Texture from Mammography and Association with Risk of Breast Cancer," was published May 13 in the journal Radiology.
Despina Kontos, PhD, is also vice chair of artificial intelligence and data science research in the Department of Radiology at Columbia University Vagelos College of Physicians and Surgeons, founding director of Columbia University's Center for Innovation in Imaging Biomarkers and Integrated Diagnostics (CIMBID), director of biomarker imaging at NewYork-Presbyterian Hospital, and chief research information officer for Columbia University Irving Medical Center. She has additional academic appointments in the Departments of Biomedical Informatics and Biomedical Engineering at Columbia University.
The two other co-senior authors are: Karla M. Kerlikowske, MD, University of California, San Francisco; and Celine M. Vachon, PhD, Mayo Clinic.
All other author are: Stacey J. Winham (Mayo Clinic); Anne Marie McCarthy (University of Pennsylvania); Christopher G. Scott (Mayo); Aimilia Gastounioti (Washington University in St. Louis); Hannah Horng (Penn), Aaron D. Norman (Mayo); Walter C. Mankowski (Penn and Columbia); Lauren Pantalone (Penn); Matthew R. Jensen (Mayo); Raymond J. Acciavatti (Penn); Andrew D. A. Maidment (Penn); Eric A. Cohen (Penn); Kathleen R. Brandt (Mayo); and Emily F. Conant (Penn).
The research was supported through by the U.S. National Institutes of Health (grants R01CA161749, P01CA154292, R01CA207084, R01CA177150) and financial support from Mayo Clinic Cancer Center.
There are no competing interests reported by any of the authors.