Rice University computer scientist Anastasios Kyrillidis has won a National Science Foundation CAREER Award to explore the theory and design of non-convex optimization algorithms, an increasingly important class used in machine learning and artificial intelligence.
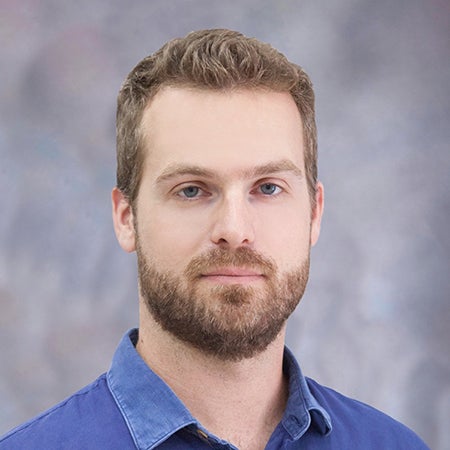
CAREER Awards are among the most competitive given by the NSF, which awards only about 500 each year across all disciplines. The awards include five-year grants to support "early career faculty who have the potential to serve as academic role models in research and education and to lead advances in the mission of their department or organization."
Kyrillidis, Rice's Noah Harding Assistant Professor of Computer Science, joined the university in 2018. His CAREER research will focus on devising technology for faster, more robust non-convex optimization algorithms.
"Non-convex optimization is at the heart of many engineering applications, especially through the wave that machine learning and artificial intelligence have triggered: physics, health care, biology, software engineering, chemistry and materials science, among others," he said.
"Given that we lack theory, more often than not, in non-convex optimization, researchers often follow trial-and-error procedures, which leads to heuristics," Kyrillidis said. "Deciding when heuristics are provable algorithms is a pressing need for the scientific community and society as a whole."
His goal is to devise algorithmic foundations and theory that will accelerate problem solving. This would include the design of fast algorithms as an active research area in machine learning, information processing and optimization research.
Understanding how performance is obtained using efficient algorithms is essential to designing practical and safely applicable learning, he said. His research will focus on three priorities: Faster convergence in structure-rich problems, with emphasis on matrix-factorized machine learning problems; algorithmic acceleration in non-convex scenarios, with a focus on neural network architectures; and acceleration techniques in machine-learning systems.
Kyrillidis earned his Ph.D. from the Swiss Federal Institute of Technology in Lausanne in 2014 prior to working as a Goldstine Postdoctoral Fellow at IBM's T.J. Watson Research Center in Yorktown Heights, New York, and as a Simons Foundation pPostdoctoral researcher at the University of Texas at Austin.