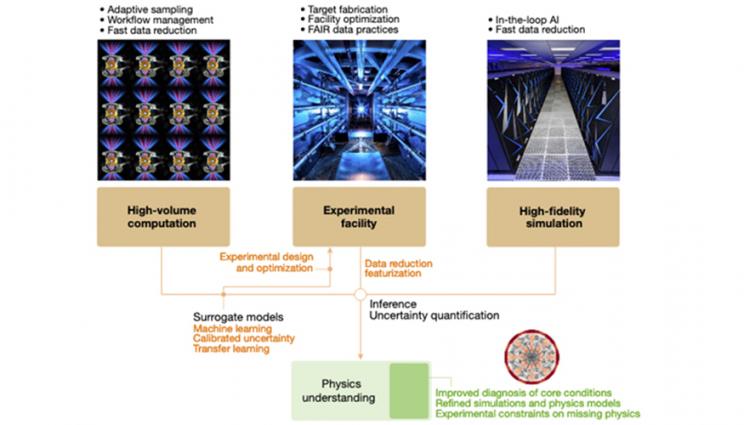
The LLNL understanding of inertial confinement fusion implosion physics is based on a combination of high-volume, lower-fidelity simulation ensembles; sparse, difficult-to-diagnose experiments; and best-physics simulations that push the limits of high-performance computing technology. Creating and synthesizing these data into an improved understanding of the physics will require multiple complementary techniques from data science, uncertainty quantification and artificial intelligence. Inset images are courtesy of Damien Jemison/LLNL.
By applying modern machine learning and data science methods to "extreme" plasma physics, researchers can gain insight into our universe and find clues about creating a limitless amount of energy.
In a recent perspective published in Nature, Lawrence Livermore National Laboratory (LLNL) scientists and international collaborators outline key challenges and future directions in using machine learning (ML) and other data-driven techniques to better understand these extreme conditions that potentially pave the pathway to nuclear fusion as an industrial power source, as well as helping to improve our understanding of the universe.
Extreme plasma is described as the physics of matter at extreme densities, temperatures and pressures like those found in the interior of stars and planets.
"Extreme plasma physics experiments historically had a very low data rate, but future planned laser facilities will have a very high shot rate, with the potential to produce huge amounts of data," said LLNL physicist Gemma Anderson, one of the lead authors of the paper. "This in turn will move the field into the big-data regime and create a corresponding need to leverage modern data science methods to a much greater extent."
The newest generation of extreme physics facilities can perform experiments multiple times a second (as opposed to almost daily) - moving away from human-based control toward automatic control. To make the most of the emerging opportunities, the team proposed a playbook for using ML in high energy density science through research design, training, best practices and support for synthetic diagnostics and data analysis.
The study of plasma physics under extreme temperatures, densities and electromagnetic field strength is important to understand astrophysics, nuclear fusion and fundamental physics. These systems are highly non-linear and are very difficult to understand theoretically or demonstrate experimentally.
Anderson and colleagues have suggested that machine learning models and data-driven methods could be the answer by reshaping exploration of these extreme systems that have proven far too complex for human researchers to do on their own. Interpreting the data from the experiments of these systems, such as the National Ignition Facility, requires simultaneously comprehending large amounts of complex multi-modal data from multiple different sources. The image above shows a potential workflow that fully integrates data-driven and machine-learning methods to achieve this goal. Optimizing extreme physics systems requires fine-tuning over large numbers of (often highly correlated) parameters. Artificial intelligence methods have proved highly successful at teasing out correlations in large datasets and can be crucial to understand and optimize systems that up to now have been difficult to understand.
The paper was a result of a workshop organized by Anderson, her LLNL colleauge Jim Gaffney and Peter Hatfield from the University of Oxford, held at the Lorentz Center in The Netherlands in January 2020. A key goal of the meeting was to write a white paper detailing the conclusions of the meeting: what standards the community should adopt, what machine learning can do for the field and what the future may hold.
Anderson said the paper will be circulated to key funding bodies and policy makers in research councils and national labs.
Lead authors of the paper were Hatfield, Gaffney and Anderson. Co-authors include Suzanne Ali, Bogdan Kustowski, Michael MacDonald, Derek Mariscal, Madison Martin and Luc Peterson from LLNL, and others from the University of York, Heslington, UK; Nikhef, National Institute for Subatomic Physics, Netherlands; DIFFER - Dutch Institute for Fundamental Energy Research, Netherlands; Instituto de Plasmas e Fusão Nuclear, Instituto Superior Técnico, Portugal; Sandia National Laboratories; Imperial College London, UK; Queen's University Belfast, Ireland; University of Rochester; Dutch National Center for Mathematics and Computer Science, Netherlands; and AWE Plc, UK.
The workshop and paper were funded by the Laboratory Directed Research and Development program.