Autonomous mobility already exists… to some extent. Building an autonomous vehicle that can safely navigate an empty highway is one thing. The real challenge lies in adapting to the dynamic and messy reality of urban environments.
Unlike the grid-like streets of many American cities, European roads are often narrow, winding and irregular. Urban environments have countless intersections without clear markings, pedestrian-only zones, roundabouts and areas where bicycles and scooters share the road with cars. Designing an autonomous mobility system that can safely operate in these conditions requires more than just sophisticated sensors and cameras.
It's mostly about tackling a tremendous challenge: predicting the dynamics of the world, in other words, understanding how humans navigate within given urban environments. Pedestrians, for example, often make spontaneous decisions such as darting across a street, suddenly changing direction, or weaving through crowds. A kid might run after a dog. Cyclists and scooters further complicate the equation, with their agile and often unpredictable maneuvers.
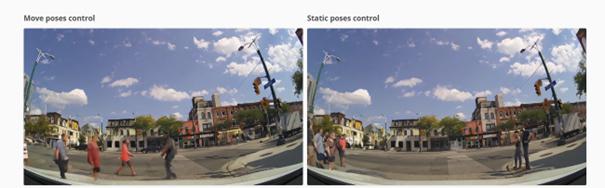
"Autonomous mobility, whether in the form of self-driving cars or delivery robots, must evolve beyond merely reacting to the present moment. To navigate our complex, dynamic world, these AI-driven systems need the ability to imagine, anticipate, and simulate possible futures-just as humans do when we wonder what might happen next. In essence, AI must learn to wonder", says Alexandre Alahi, head of EPFL's Visual Intelligence for Transportation Laboratory (VITA).
Pushing the boundaries of prediction: GEM
At VITA laboratory, the goal of making AI "wonder" is becoming a reality. This year, the team has had seven papers accepted to the prestigious Conference on Computer Vision and Pattern Recognition (CVPR'25). Each contribution introduces a novel method to help AI systems imagine, predict, and simulate possible futures-from forecasting human motion to generating entire video sequences. In the spirit of open science, all models and datasets are being released as open source, empowering the global research community and industry to build upon and extend this work. Together, these contributions represent a unified effort to give autonomous mobility the ability not just to react, but to truly anticipate the world around them.
One of the most innovative models is designed to predict video sequences from a single image captured by a camera mounted on a vehicle (or any egocentric view). Called GEM (Generalizable Ego-Vision Multimodal World Model), it helps autonomous systems anticipate future events by learning how scenes evolve over time.
As part ot the Swiss AI Initiative, and in collaboration with four other institutions (University of Bern, SDSC, University of Zurich and ETH Zurich), they trained their model using 4000 hours of videos spanning autonomous driving, egocentric human activities (meaning, activities from the first person point of view) and drone footage. GEM learns how people and objects move in different environments. It uses this knowledge to generate entirely new video sequences that imagine what might happen next in a given scene, whether it's a pedestrian crossing the street or a car turning at an intersection. These imagined scenarios can even be controlled by adding cars and pedestrians, making GEM a powerful tool for safely training and testing autonomous systems in a wide range of realistic situations.
To make these predictions, the model looks simultaneously at several types of information, also called modalities. It analyzes RGB images-which are standard color video frames-to understand the visual context of a scene, and depth maps to grasp its 3D structure. These two data types together allow the model to interpret both what is happening and where things are in space. GEM also takes into account the movement of the camera (ego-motion), human poses, and object dynamics over time. By learning how all of these signals evolve together across thousands of real-world situations, It can generate coherent, realistic sequences that reflect how a scene might change in the next few seconds.
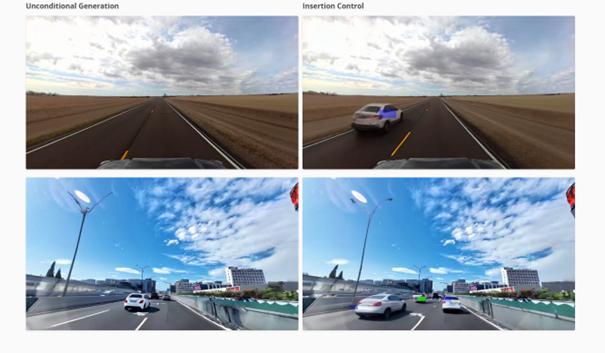
"The tool can function as a realistic simulator for vehicles, drones and other robots, enabling the safe testing of control policies in virtual environments before deploying them in real-world conditions. It can also assist in planning by helping these robots anticipate changes in their surroundings, making decision-making more robust and context-aware," says Mariam Hassan, Ph.D student at VITA lab.
The road to predictions
Predicting human behavior is a complex and multi-faceted challenge, and GEM represents just one piece of the VITA Lab's broader effort to tackle it. While GEM focuses on generating the videos of the future and exposing autonomous systems to diverse virtual scenarios, other research projects from Professor Alahi's team are tackling lower levels of abstractions to enhance prediction with robustness, generalizability, and social awareness.
For example, one of them aims to certify where people will move, even when the data is incomplete or slightly off. Meanwhile, MotionMap tackles the inherent unpredictability of human motion through a probabilistic approach. This probabilistic approach helps systems prepare for unexpected movements in dynamic environments.
These efforts form a comprehensive framework that maps out the complex interactions at play in crowded urban settings. There are still challenges: long-term consistency, high-fidelity spatial accuracy, and computational efficiency are still evolving. At the heart of it all lies the toughest question: how well shall we predict people who don't always follow patterns? Human decisions are shaped by intent, emotion, and context-factors that aren't always visible to machines.
Launched in December 2023 by EPFL and ETH Zurich, the Swiss AI Initiative is supported by more than 10 academic institutions across Switzerland. With over 800 researchers involved and access to 10 million GPU hours, it stands as the world's largest open science and open source effort dedicated to AI foundation models. The model developed by VITA lab, in collaboration with four other institutions (University of Bern, SDSC, University of Zurich and ETH Zurich) is among the first major models to emerge from this ambitious collaboration. It was trained on the Alps supercomputer at the Swiss National Supercomputing Centre (CSCS), which provided the massive computational power needed to process vast amounts of multimodal data.
In Switzerland, fully autonomous mobility is not yet permitted on public roads. However, as of March 2025, cars equipped with advanced assisted driving systems will be allowed to steer, accelerate and brake autonomously. While the drivers must remain alert and ready to take control, this marks a significant step towards everyday automation. Cantons have the authority to approve specific routes for fully autonomous vehicles, operating without a human on board and monitored remotely by control centers. These routes will primarily be used by buses and delivery vans.